CabAnon visualised
As described in our previous article, two visualizations have been created in order to map taxi rides. The first one displays the original dataset published by the city of New York, and the second one represents the anonymised datasets.
An accurate map of taxis locations
The data initially published by New York City include the time-stamped coordinates (longitude and lattitude) of each taxi across the day. We decided to represent each of them with a dot on the city map in order to identify high density zones.
A chloropeth map of taxis
In the anonymised datasets (article 29 working Party or Uber) a taxi's location corresponds to a Zip Code Tabulation Area (ZCTA). As a result, the number of taxis present in each ZCTA at any given time is calculated and then correlated with a color scale which is updated for each selected time slot. The traffic density is visualised globally, allowing comparisons between ZCTAs.
A matrix of the possible data uses
We imagined several use case scenarios to explore different ways of using these datasets in order to assess the usefulness of anonymised datasets. One of those scenarios is developed below.
Those scenarios are mapped on a two-axis matrix according to the features of the required data. The horizontal axis (anonymity/granularity) corresponds to the accuracy of the data. The more granular the data, the more accurate the information they provide on trips are, and therefore the more they can affect one's privacy. The vertical axis (combined/ autonomous) represents the extent to which the New York City taxis dataset can be used indepedently of any other one.
Examples of possible use of such data include:
- Prioritizing public resources, for example by triggering field investigation to better understand the dynamics of urban flows. The purpose being to identify which areas to study, anonymised data are sufficient to fulfill it;
- Urban planning, including refurbishing public roads according to the effective presence of vehicules. As explained in our Innovation and Foresight review dedicated to the smart city, redevelopment of public spaces, in particular roadworks, requires the availability of fairly accurate geolocalised data (at least at street level);
- Improving the traffic flow, by identifying high traffic density areas. If you want to make the traffic more fluid, you will need in many cases some fairly accurate geolocalised and time-stamped data (at least at street level and every few minutes);
- Developing new services, for example by stimulating the creation of alternative or additional transport offers (like shuttle services, carpooling ...). The goal is in this case to understand the existing transport environment in order to implement a new service. There is therefore no need to have accurate data, even though adding other (anonymised) datasets can be relevant according to the type of service being created;
- Improving "on demand" services by seeking to reduce user waiting time for on-demand vehicles. In this case, it could be useful to have some really accurate data, close to real time.
Exploring possible use of the New-York City taxis public dataset
Creating new transportation services
Context
Let's imagine we are creating a new transportation service in New-York City. Given that competition is tough, it is necessary to find a niche market in which the service would be established. With this in mind, it is possible to opt for a service located on the edge of public transportation services, in order to complement and enhance an already well-established transportation network. We may for example consider creating a shuttle service which provides micro-trips from a public transportation station to a person's home (
note that Uber offers a similar service in Florida). If you want to know where to implement such service you will need to :
- identify and qualify (location, time, capacity...) the use of alternatives to the public system, for example the use of taxis ;
- understand how public transports are used (station activity, flows);
- compare the use of different types of transportations and understand their overlaps and differences;
Understanding the taxis distribution
The chloropleth map can help for the first point, over 30 minutes time slots. For the other two, it would be necessary to add datasets from New-York’s subway, so that we can compare both datasets. Since this is not implemented in our visualisation, we made the comparison using the NYC public transport map distributed by the
MTA.
The visualisation enables the identification of the time slots of little interest for our service. Typically, between 5.30am and 9.30am the number of available taxis is low (less than 150 taxis in high density areas).
2am is one of the busiest hours. Most taxis taking passengers in Manhattan stay on the island. However, in the two most crowded areas of Manhattan, Union Square (ZCTA 10003) and Lexington Avenue (ZCTA 20022), some taxis are going to Brooklyn and Queens (both neighboroods are poorly served in terms of public transportation compared to Manhattan). Besides, public transports are circulating on periods of 30 minutes, so it would be easy to determine at what time users will exit the public transportation system.
In order to place the shuttle service strategically, it would be highly recommended to operate near the following stations between 2am and 3am:
- 46 Street Station (11103): line E, M and R
- Bedford Avenue (11211): line L
In this case, we see that it is possible to use anonymised data to understand a specific environment. The same exercise can probably be done with the original raw data, but their higher level of granularity would not necessarily improve the stations' choice. Indeed, it is more relevant to know the average taxi density in an area, and know their trip from one area to another rather than their precise location and route.
Anonymizing a dataset, especially with the methods presented in this article[HE1] [CR2] does not necessarily mean that the data become useless. Some innovative solutions are emerging to further improve the confidentiality/usability ratio: differential privacy, co-clustering, etc., which will be presented in future articles.
Privacy, a matter of how the data are used?
We have shown that anonymised datasets can be useful and relevant to meet some specific goals when setting up a product or a service. Nevertheless, some applications require a high level of granularity that could undermine the privacy of individuals. If the purpose of a service or product determines the corresponding level of anonymity for the dataset, woudn't it be possible to imagine and implement new ways of sharing and distributing (open) data that foster both innovation while protecting privacy? We propose such possibilities in our report The Platform of a City (La Plateforme d’une ville) where we explore four scenarios and as many modalities that could allow public actors to have access to the high quality datasets they need.
In the case of the open data related to New York City's taxis, the controversies arose from their poor anonymisation whereas they can be accessed by anyone, including individuals with questionable motivations. When publishing a dataset, one must therefore strike the right balance between anonymisation techniques and data access. Thus data access could be associated with rules when reused such as specific limitations (on purposes and uses) or security measures to be implemented (such as pseudonymisation).
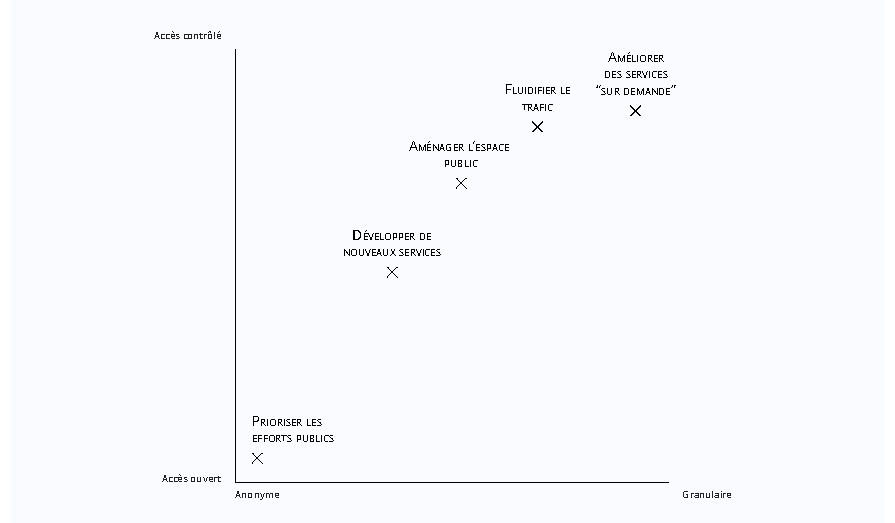
Our previous examples show that an on-demand service based on very accurate time-stamped data might have access to pseudonymised datasets, provided that adequate security measures are in place, and that this data processing is in full compliance with the General Data Protection Regulation (GDPR). In the case of anonymised datasets (as understofood in the GDPR, and following the article 29 working party’s opinion), it would be possible to publish and give free access to it, without any conditions or specific protection.
CabAnon is an open source project
You can contribute to Cabanon on our
Linc's Github, and have a look on our other projects (Cookieviz, delisting...).