Y.A. de Montjoye and A. Gadotti: “Moving beyond de-identification will allow us to find a balance between using data and preserving people’s privacy"
Rédigé par Félicien Vallet
-
13 juin 2019Following their visit to our laboratory, LINC interviewed Yves-Alexandre de Montjoye and Andrea Gadotti about the challenges raised by data anonymisation and ways to find a balance between using data and protecting people’s privacy.
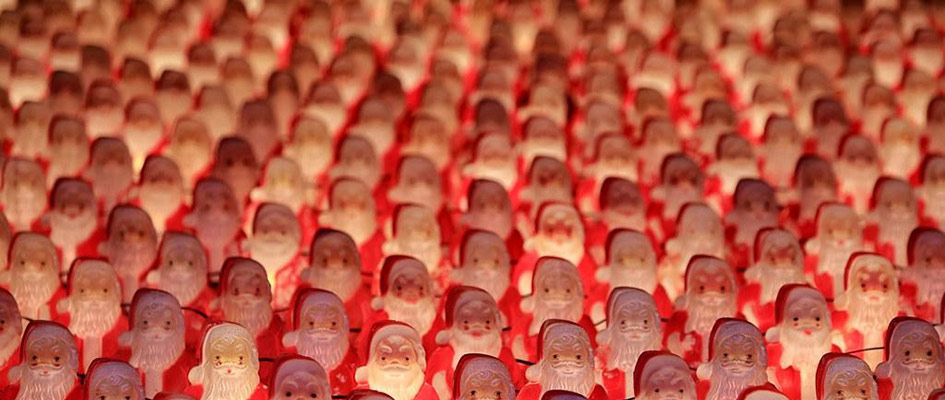
LINC: You are researchers on the subject of privacy with a focus on anonymization. This notion seems to have evolved over time. Could you tell us why, and how you would define it today?
The goal of anonymization is to allow organizations and companies to use and share data without endangering individual’s privacy. The concept is good and easy to understand: “your data will be used anonymously, e.g. for statistical purposes, and will not be linked back to you”.
Historically, anonymization has been achieved through de-identification. De-identification is a release-and-forget approach: direct identifiers are removed from the dataset and indirect identifiers are blurred, suppressed, etc. The dataset is then released to analysts, possibly as open data.
Human activities are increasingly being monitored for various reasons. Given the existence of such records about our lives, is it still possible to remain anonymous?
Nowadays, most of our daily activities generate digital data “automatically”. Whenever we make a call, go to work, search on Google, pay with our credit card, we generate data. While de-identification might have worked in the past, it doesn’t really scale to the type of large-scale datasets being collected today.
In 2013, we also proposed a metric which we called unicity, to quantify how much information is needed to re-identify a user in large-scale behavioral datasets. Using unicity, we showed that 4 random points (i.e. time and location where a person has been) are enough to uniquely identify someone 95% of the time in a dataset with 1.5 million individuals. We then extended the study to credit card data, obtaining similar results and showing that some people’s shopping habits are more unique than others’. Since then, numerous research papers have used unicity to study the re-identification risks of big data datasets.
All these results lead us to conclude that an efficient enough, yet general, anonymization method is extremely unlikely to exist for high-dimensional data. This opinion was shared by the [US] President's Council of Advisors on Science and Technology (PCAST), which stated that de-identification “remains somewhat useful as an added safeguard, but it is not robust against near‐term future re‐identification methods”.
You work on an initiative called OPAL. Could you describe to us what it is about?
Location data has a lot of potential for good, especially in developing countries. Orange’s Data for Development (D4D) challenges and other work showed how mobility data could be used to reduce crime, estimate poverty or assess the impact of natural disasters in real-time. However, the D4D challenges and others were one-off initiatives: excellent to prove the potential but, to have a deep and long-lasting impact on the country, we had to find sustainable ways for National Statistical Offices and other organizations to safely access the data. OPAL aims to be a solution to this problem.
OPAL is a query-based system that moves away from the release-and-forget model. In practice, this means that individual-level data is never shared with the analysts. The sensitive data are stored on a protected remote server, but analysts can send queries to analyze them. OPAL ensures that the response to each query is aggregated over many users, so that the analyst cannot see which data relate to which individual.
Practically, how does OPAL protect the privacy of individuals whose data are being processed?
OPAL enforces aggregation for every analysis. This greatly reduces the risks of sharing individual-level data. However, it has long been shown that query-based systems can still be vulnerable to a range of re-identification attacks. A malicious analyst can often submit a series of seemingly innocuous queries whose outputs, when combined, will allow them to infer private information about participants in the dataset.
Building a practical system that is useful and protects against all possible attacks is extremely challenging and still an open research question. OPAL takes a practical, layered, approach to protect privacy using a combination of technical measures (e.g. noise addition, query suppression, auditing and rate limiting) along with a range of governance tools such as the vetting of partners and algorithms by an ethics board, full logging and monitoring of the queries, etc.
Anonymisation is often being described as an innovation killer. How projects such as yours can change this perception?
Well, it really depends what you mean by “anonymization”. If de-identification was the only way to anonymize data, we’d indeed have a big problem.
Thankfully, it’s not, and it is actually less and less used. Modern privacy engineering approaches combine state-of-the-art privacy-enhancing technologies with computer security and data governance best practices. We recently published a white paper on this in the context of AI: Solving AI’s privacy problem.
Such integrated, and often adversarial, approaches are absolutely crucial: we need to move beyond de-identification and start using modern solutions to unlock the huge potential of data for social good and economic development. Else, we risk being stuck in the false dichotomy that we have either innovation or privacy.
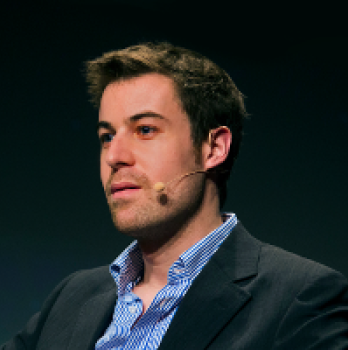
Yves-Alexandre de Montjoye
Yves-Alexandre de Montjoye is an Assistant Professor at Imperial College London where he leads the Computational Privacy Group and a Special Adviser to EC Commissioner Margrethe Vestager. He is affiliated with the Data Science Institute and Department of Computing.
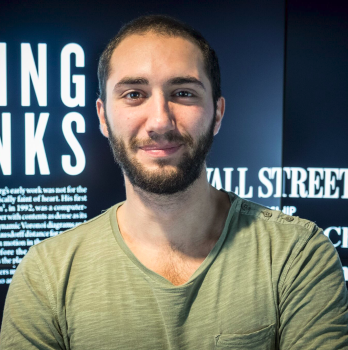
Andrea Gadotti
Andrea Gadotti is a PhD student in Computational Privacy at Imperial College London.