Paul Francis: “We can achieve very good anonymity and still provide useful analytics”
Rédigé par Félicien Vallet
-
05 juin 2018Following his visit to our laboratory in May 2018, LINC proposed an interview with Paul Francis about the challenges raised by data anonymization and the innovative way he developed to tackle them.
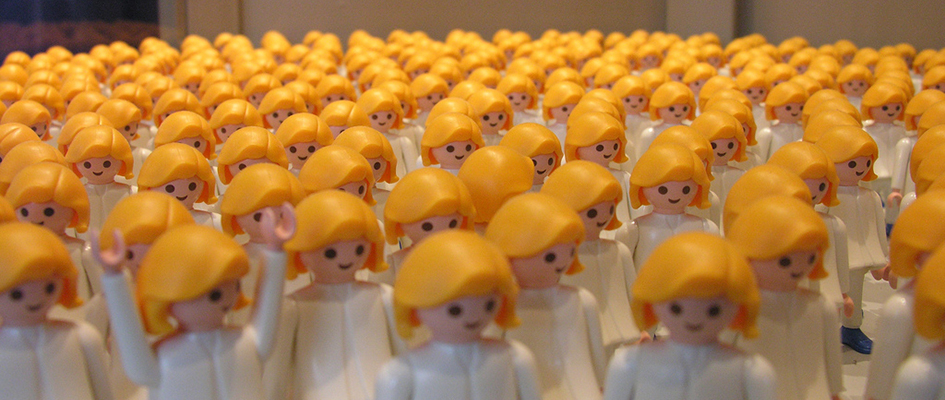
LINC : While most people believe that anonymization is an easy task for which you only need to discard direct identifiers, most experts argue that it is almost impossible to anonymise a dataset, given the increase in computational power, the quantity of external data available and the development of new mathematical tools and methods. What is your take on this issue?
Paul Francis : Data anonymization has a long history of failure. Some of these failures, like with AOL and Netflix, come from this non-expert group you mention. But there are also a lot of failures from within the academic community, where for instance a formal model like K-anonymity turns out to have unexpected vulnerabilities. I think that the academic community has become very fearful of failure, to the point where they have retreated to the differentially private measure of anonymity in which every release of new data results in additional privacy loss. This produces mechanisms that have very little practical utility. The diversity of new ideas coming out of the academic community has shrunk to almost nothing. I know researchers who firmly believe that mechanisms based on the differentially private measure is the only legitimate way forward.
I believe that there are many new and innovative ideas still to be developed. In order to explore them, however, we need to be prepared to live with a certain amount of uncertainty and occasional setbacks. We need to find creative ways to foster open and practical evaluations of anonymity systems. Ideas like the PWS CUP anonymization competition in Japan.
You developed the Diffix anonymization method that is implemented by your spin-off Aircloak. It is especially designed to deal with dynamic datasets and ensure anonymization is maintained over time. What are the main differences between static and dynamic anonymization?
Aircloak is not so much a “spin-off” as a “spin-with”. From the beginning, Aircloak has been a full research partner in the development of Diffix and the technologies that preceded it. In fact, five years ago we started with a system based on a differentially private measure, and proceeded through a couple more rejected technologies before ending with Diffix. During these years, Aircloak benefitted tremendously from the support of the German government through the EXIST program.
For us, there is no such thing as static data. There is just a continuum from less dynamic (i.e. census data updated every 10 years) to more dynamic (a real-time stream of geolocation data). We chose early on to base our system on query-by-query anonymization rather than full-database anonymization. This was, however, not so much to deal with dynamic data per se but rather because a query-by-query approach gives the analyst more flexibility, and allows us to minimize distortion.
To guarantee your customers the quality of the data anonymization you recently launched a bounty program. Could you elaborate on its goal and organisation?
First of all, I prefer to avoid the word “guarantee”. Especially among academics, it implies a mathematical certainty that nothing can go wrong. In order to provide good analytic utility, Diffix is necessarily too complex for mathematical guarantees. At the same time, Data Protection Authorities (DPA) like CNIL lack either the time or the expertise to fully certify systems as general as Diffix. We ourselves are of course also not perfect and cannot guarantee that we have found every possible vulnerability.
The Aircloak bounty program helps us discover new vulnerabilities in Diffix in a controlled setting. It allows us to patch vulnerabilities before they become known to the public. Over time, it increases the strength of Diffix, and gives us, our customers, and DPAs confidence in the system.
We are the only group that has ever launched a bounty program for anonymity. I can tell you that we were quite nervous about it --- not so much that someone would find a fundamental flaw --- if that happens then better to learn sooner than later. Rather, we were concerned that a minor and fixable flaw would be over-reported and the reputation of Diffix would be wrongly damaged. But of course that can happen with or without a bounty program, so we felt it best to push ahead.
The bounty program is a “white box” setup, where the attacker has all of the details of how Diffix operates. We provide a variety of real datasets that can be attacked, or the attacker can provide his or her own. The goal of any attack is to violate one of the three criteria for anonymity as suggested by the EU Article 29 Working Party opinion: singling-out, linkability, and inference. Depending on the level of success of the attack, the attacker may receive up to $5000.
A bounty program is only as good as its attackers. Can you tell us how it went and what knowledge you obtained from the successful attacks?
The first phase of the attack lasted 6 months, from December 2017 through May 2018. We have published various statistics about the attackers so that people can see how the program went. In the end, 35 attackers requested accounts from such places as Aarhus Univ, Budapest Univ, EPFL, Georgetown Univ, INRIA, MIT, Univ College London, Univ Southern California, and Univ Quebec as well as a number of companies. Over 30 million attack queries in all were issued.
There were two successful attacks, one based on singling out, and one based on linkability. Both attacks were statistical in nature, taking the results of hundreds of queries and applying solvers or machine learning. We know how to modify Diffix to defend against both, and the patches are under development. The first phase of the program was very valuable to us. We learned about a class of attacks that wasn’t on our radar, and can fix them before there is any real risk to our customers. We plan a second phase of the program after the next major release later in the year.
It seems that we are torn between the necessity to protect privacy and the will to extract knowledge from datasets that could be used for public interest. If you had to take a guess on how we’ll deal with these issues in the future what would it be?
The tension between privacy and extracting knowledge will always be with us. Our experience in the last few years however suggests that we can achieve very good anonymity and still provide quite useful analytics for a wide range of use cases. Our focus until now has been on use-case generality: that is, providing a tool that works equally well for all use cases (banking, location, transportation, medical, etc.). For the near future, we will remain general while we expand beyond basic statistics into advanced analytics and machine learning. But after that, I can envision drilling down into specific use cases to really improve utility in the context of specific types of data or business cases while staying anonymous.
More generally, I hope that our bounty program can serve as a template for other anonymization technologies and other companies. It would be really fantastic if industry, academia, and government can move towards a common scoring system and toolkit for evaluating and improving anonymity.
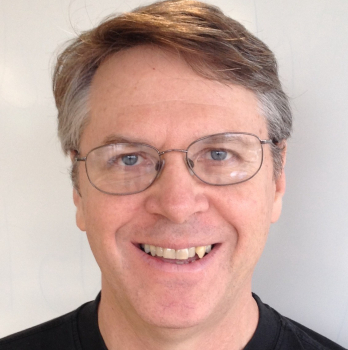
Paul Francis
Paul Francis is a scientific director at the Max Planck Institute for Software Systems in Kaiserslautern and Saarbrücken Germany and co-founder of the startup Aircloak. His research focuses on data anonymity.
Illustration : Playmobil Army - Flickr - cc-by Dsungi